class: center, middle, inverse, title-slide # Confounding ## What If: Chapter 7 ### Elena Dudukina ### 2021-02-04 --- # Confounding ## Lack of exchangeability .center[ π§ ] .center[ **βοΈ** **βοΈ** ] .pull-left[ πΏ πΏ πΏ πΏ πΏ πΏ πΏ πΏ πΏ πΏ πΏ πΏ πΏ πΏ πΏ πΏ πΏ πΏ πΏ πΏ πΏ πΏ πΏ πΏ πΏ πΏ πΏ πΏ πΏ πΏ πΏ πΏ πΏ πΏ πΏ πΏ πΏ πΏ πΏ πΏπΏ πΏ πΏ πΏ πΏ πΏ πΏ πΏ πΏ πΏ πΏ πΏ πΏ πΏ πΏ πΏ πΏ πΏ πΏ πΏ πΏ πΏ πΏ πΏ πΏ πΏ πΏ πΏ πΏ πΏ πΏ πΏ πΏ πΏ πΏ πΏ πΏ πΏ πΏ πΏ πΏ πΏ πΏ πΏ πΏ πΏ πΏ πΏ πΏ πΏ πΏ πΏ πΏ πΏπΏ πΏ πΏ πΏ πΏ πΏ πΏ πΏ ] .pull-right[ π π π π π π π π π π π π π π π π π π π π π π π π π π π π π π π π π π π π π π π π π π π π π π π π π π π π π π π π π π π π π π π π π π π π π π π π π π π π π π π π π π π π π π π π π π π π π π π π π π π π π π ] .center[ **?** ] --- # 7.1 The structure of confounding ## Bias due to common causes - A: treatment - Y: outcome - L: confounder (a common cause of A and Y) ## Paths between A and Y - A β‘οΈ Y: causal effect (directed path) - A β¬ οΈ L β‘οΈ Y: biasing backdoor path (starts with a head pointing into A) * The associational risk ratio `\(\frac{Pr[Y=1|A=1]}{Pr[Y=1|A=0]}\)` is not causal risk ratio `\(\frac{Pr[Y^a=1]}{Pr[Y^a=0]}\)` 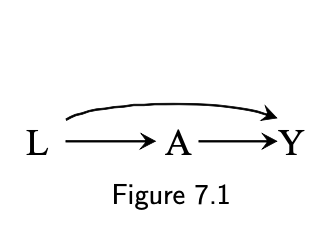 --- ## 7.1 Examples .pull-left[ Occupational factors (healthy worker bias) - A: working as a firefighter - Y: mortality - L: being fit to work as a firefighter Confounding by indication (channeling bias) - A: drug (aspirin) - Y: mortality - L: indication for the drug (heart disease) - U: (unmeasured) atherosclerosis ] .pull-right[ 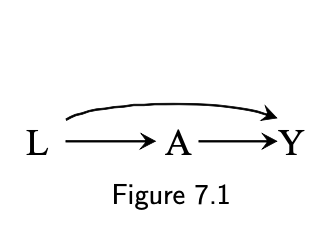 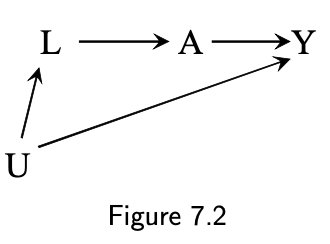 ] --- .pull-left[ Lifestyle - A: behavior (exercise) - Y: mortality - L: lifestyle (smoking) - U: (unmeasured) personality/socioeconomic determinants Reverse causation - A: behavior - Y: clinical disease - L: lifestyle (smoking) - U: (unmeasured) subclinical disease ] .pull-right[ 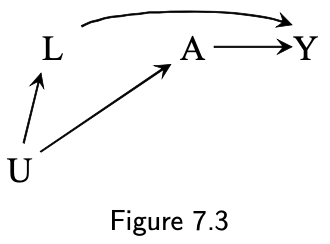 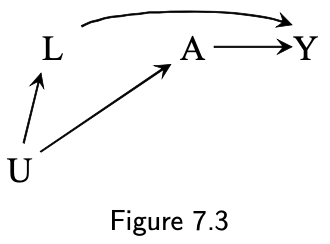 ] --- Genetic factors (linkage disequilibrium) - A: DNA sequence - Y: developing a trait - L: DNA sequence confounding effect of A if L and A are often being inherited together and L causes Y - U: ethnicity Social factors - A: income at age 65 - Y: disability at age 75 - L: disability at age 55 - Time-varying flavor Environmental exposures - A: airborne particle - Y: coronary heart disease - L: other pollutants, which levels co-vary together with A - U: (unmeasured) weather (controls the level of all types of airborne pollutants) --- ## Assumptions for the remainder of the chapter - No selection bias (Chapter 8) - No measurement bias (Chapter 9) - No random variabiity (Chapter 10) --- # 7.2 Confounding and exchangeability ## Assuming consistency and positivity hold - Exchangeability holds `\(Y^a \perp\perp A\)`: potential outcome under the treatment regime is independent from actually observed treatment for all treatment levels (perfect randomization) -- - When `\(Y^a \perp\perp A\)` is true and treatment is binary - the average causal effect `\(E[Y^{a=1}] - E[Y^{a=0}]\)` equals the observed associational effect `\(E[Y|A=1] - E[Y|A=0]\)` -- - When *conditional* exchangeability holds `\(Y^a \perp\perp A|L\)`, the verage caussal effect is identifiable - `\(E[Y^{a=1}] - E[Y^{a=0}] = \Sigma_{l}E[Y|L=l, A=1]*Pr[L=l] - \Sigma_{l}E[Y|L=l, A=0]*Pr[L=l]\)` - G-methods (IPTW, standardization) for marginal estimates --- # Is there a set of variables L that guarantees conditional exchangeability holds? ## No guarantees (unless well-conducted RCT) * Use of DAGs to show the data-generating mechanism and assumptions * No residual confounding (conditional exchangeability) assumption * Backdoor criterion (J. Pearl, 1995) - No node in adjustment set is a descendant (child) of exposure (not a mediator) - The adjustment set blocks all paths pointing into exposure (blocks all backdoor paths) - Consider magnitude and te direction of bias by unmeasured confounding --- # 7.3 Confounding and the backdoor criterion .pull-left[ Adjustment sets sufficient to eliminate **confounding**: - 7.2: {L}, {U} - 7.3: {L}, {U} - 7.4: {} (empty set) - adjusting for L opens a biasing path - A β¬ οΈ U2 β‘οΈ [L] β¬ οΈ U1 β‘οΈ Y - think **confounding**, not **confounders** - role of the variable changes when other variables are adjusted ] .pull-right[ 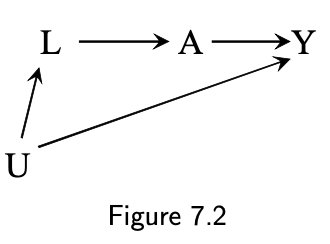 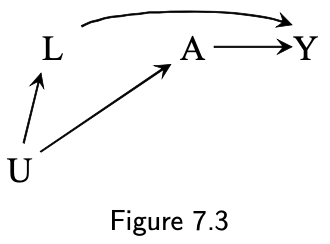 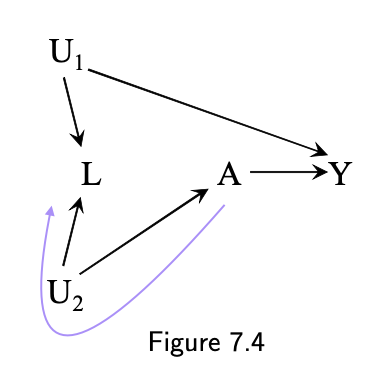 ] --- .pull-left[ Fig 7.5 - A β¬ οΈ L β¬ οΈ U1 β‘οΈ Y (open biasing path, L is not a collider) - A β¬ οΈ U2 β‘οΈ L β¬ οΈ U1 β‘οΈ Y (biasing path is closed with the collider L) - {U1}, {L, U2}, {L, U1} Fig 7.6 - {L1}, {U1}, {L, L2}, {L, U2}, {L, L1}, {L, U1} ] .pull-right[ 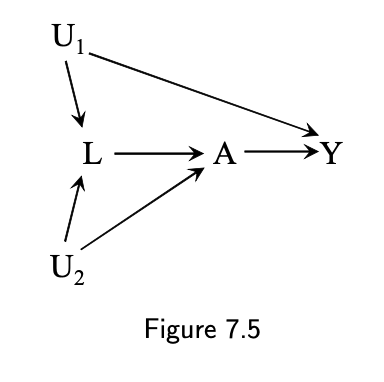 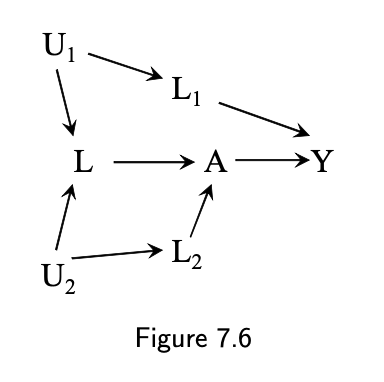 ] --- # 7.4 Confounding and confounders .pull-left[ Traditional criteria - Association with the exposure - Association with the outcome - Not on a causal pathway Change of the estimate after adjustment may occur for the reasons other than adjusting confounding (selection bias, non-collapsibility of effect measures) ] .pull-right[ 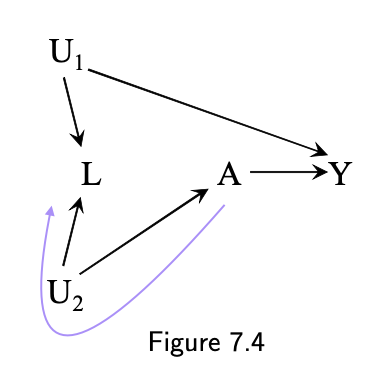 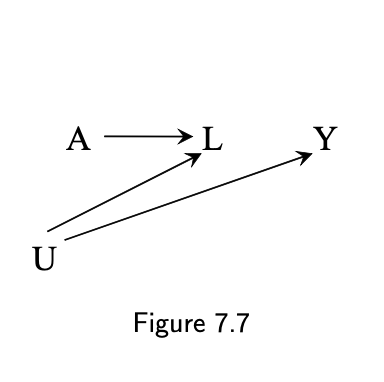 Is L a confounder? ] --- # 7.6 Confounding adjustment - Sufficient set for confounding adjustment - Methods - G-methods (marginal and conditional effects) - Standardization - IP weighting (deleting an arrow L β‘οΈ A) - g-estimation - Stratification-based methods (conditional effects only) - Stratification - Restriction - Matching --- # References HernΓ‘n MA, Robins JM (2020). Causal Inference: What If. Boca Raton: Chapman & Hall/CRC (v. 31jan21)